Abstract
This paper proposes an improved video action recognition method, primarily consisting of three key components. Firstly, in the data preprocessing stage, we developed multi-temporal scale video frame extraction and multi-spatial scale video cropping techniques to enhance content information and standardize input formats. Secondly, we propose a lightweight Inception-3D networks (LI3D) network structure for spatio-temporal feature extraction and design a soft-association feature aggregation module to improve the recognition accuracy of key actions in videos. Lastly, we employ a bidirectional LSTM network to contextualize the feature sequences extracted by LI3D, enhancing the representation capability for temporal data. To improve the model’s robustness and generalization ability, we introduced spatial and temporal scale data augmentation techniques in the preprocessing stage, effectively extracting video key frames and capturing key regional actions. Furthermore, we conducted an in-depth study on spatio-temporal feature extraction methods for video data, effectively extracting spatial and temporal information through the LI3D network and transfer learning. Experimental results demonstrate that the proposed method achieves significant performance improvements in video action recognition tasks, providing new insights and approaches for research in related fields.
Keywords
video action recognition
multi-scale preprocessing
lightweight I3D (LI3D)
spatio-temporal feature extraction
bidirectional LSTM
Data Availability Statement
The code and data supporting the findings of this study are available from the following link: https://drive.google.com/file/d/1oJMhtkD2SPBO_g5uReY5GZQvltis7-9E/view?usp=sharing.
Funding
This work was supported without any funding.
Conflicts of Interest
Fafa Wang is an employee of Beijing iQIYI Technology Co., Ltd., Beijing 100080, China.
Ethical Approval and Consent to Participate
Not applicable.
Cite This Article
APA Style
Wang, F., Jin, X., & Yi, S. (2024). LI3D-BiLSTM: A Lightweight Inception-3D Networks with BiLSTM for Video Action Recognition. IECE Transactions on Emerging Topics in Artificial Intelligence, 1(1), 58–70. https://doi.org/10.62762/TETAI.2024.628205
Publisher's Note
IECE stays neutral with regard to jurisdictional claims in published maps and institutional affiliations.
Rights and permissions
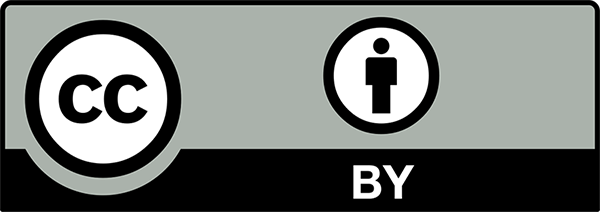
Copyright © 2024 by the Author(s). Published by Institute of Emerging and Computer Engineers. This article is an open access article distributed under the terms and conditions of the Creative Commons Attribution (CC BY) license (
https://creativecommons.org/licenses/by/4.0/), which permits use, sharing, adaptation, distribution and reproduction in any medium or format, as long as you give appropriate credit to the original author(s) and the source, provide a link to the Creative Commons licence, and indicate if changes were made.