Abstract
In the era of rapid technological advancement, the demand for sophisticated Multi-Object Tracking (MOT) systems in applications such as intelligent surveillance and autonomous navigation has become increasingly critical. However, existing models often struggle with accuracy and efficiency in densely populated or dynamically complex environments. Addressing these challenges, we introduce a novel deep learning-based MOT model that incorporates the latest CT-DETR detection technology and an advanced ReID module for improved pedestrian tracking. Experimental results demonstrate the model's superior performance in accurately identifying and tracking multiple targets across varied scenarios, significantly outperforming existing benchmarks. This research not only marks a significant leap forward in the field of video surveillance technology but also lays a foundational framework for future advancements in intelligent system applications, underscoring the importance of innovation in deep learning methodologies for real-world challenges.
Keywords
multi-object tracking
deep learning
CT-DETR
pedestrian re-identification
intelligent surveillance systems
Data Availability Statement
Data will be made available on request.
Funding
This work was supported without any funding.
Conflicts of Interest
The authors declare no conflicts of interest.
Ethical Approval and Consent to Participate
Not applicable.
Cite This Article
APA Style
Gao, M., & Yang, S. (2024). CT-DETR and ReID-Guided Multi-Target Tracking Algorithm in Complex Scenes. IECE Transactions on Emerging Topics in Artificial Intelligence, 1(1), 44–57. https://doi.org/10.62762/TETAI.2024.240529
Publisher's Note
IECE stays neutral with regard to jurisdictional claims in published maps and institutional affiliations.
Rights and permissions
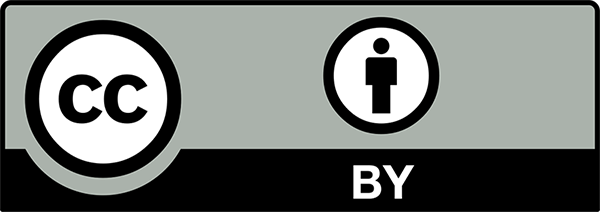
Copyright © 2024 by the Author(s). Published by Institute of Emerging and Computer Engineers. This article is an open access article distributed under the terms and conditions of the Creative Commons Attribution (CC BY) license (
https://creativecommons.org/licenses/by/4.0/), which permits use, sharing, adaptation, distribution and reproduction in any medium or format, as long as you give appropriate credit to the original author(s) and the source, provide a link to the Creative Commons licence, and indicate if changes were made.