Abstract
Interpreting NMR spectra to accurately predict molecular structures remains a significant challenge in chemistry due to the complexity of spectral data and the need for precise structural elucidation. This study introduces NMRGen, a generative modeling framework that predicts molecular structures from NMR spectra and molecular formulas. The framework combines a SMILES autoencoder (GRU-based encoder-decoder) and an NMR encoder (CNN and DNN layers) to map spectral data to molecular representations. The SMILES autoencoder compresses and reconstructs SMILES strings, while the NMR encoder processes NMR spectra to generate latent vectors aligned with those from the SMILES encoder. Experiments were conducted using NMR spectra and SMILES datasets. The model was trained in three stages: (1) training the SMILES autoencoder, (2) aligning latent vectors from the NMR encoder, and (3) simultaneous training of both components. Results revealed that while the SMILES autoencoder performed adequately, the NMR encoder struggled to map spectral data effectively. Most generated SMILES strings were invalid, with valid ones primarily consisting of carbon chains (e.g., CCC...C). The Tanimoto coefficient between generated and target molecules ranged from 0.1 to 0.2, indicating low similarity. Despite these limitations, NMRGen demonstrates the potential of generative models for molecular structure prediction. Future work will focus on improving performance through larger datasets, advanced loss functions, and enhanced architectures.
Data Availability Statement
The code used for this study is publicly available on GitHub at the following link: https://github.com/rajavavek/Predicts-Molecular-Structures-from-NMR-Spectra.
Funding
This work was supported without any funding.
Conflicts of Interest
Raja Vavekanand is an employee of Datalink Research and Technology Lab, Islamkot 69240, Sindh, Pakistan.
Ethical Approval and Consent to Participate
Not applicable.
Cite This Article
APA Style
Vavekanand, R. (2025). NMRGen: A Generative Modeling Framework for Molecular Structure Prediction from NMR Spectra. IECE Transactions on Emerging Topics in Artificial Intelligence, 2(1), 16–25. https://doi.org/10.62762/TETAI.2024.277656
Publisher's Note
IECE stays neutral with regard to jurisdictional claims in published maps and institutional affiliations.
Rights and permissions
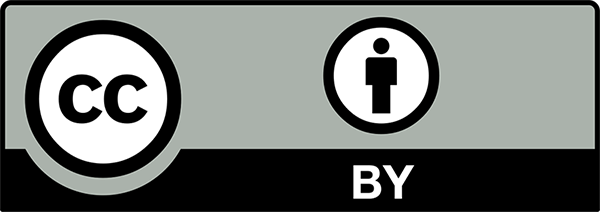
Copyright © 2025 by the Author(s). Published by Institute of Emerging and Computer Engineers. This article is an open access article distributed under the terms and conditions of the Creative Commons Attribution (CC BY) license (
https://creativecommons.org/licenses/by/4.0/), which permits use, sharing, adaptation, distribution and reproduction in any medium or format, as long as you give appropriate credit to the original author(s) and the source, provide a link to the Creative Commons licence, and indicate if changes were made.