Abstract
Recently, the primary reason for blindness in adults has been diabetic retinopathy (DR) disease. Therefore, there is an increasing demand for a real-time efficient classification and detection system for diabetic retinopathy (DR) to overcome fast-growing disease (DR). We introduced a novel deep hybrid model for auto-mated diabetic retinopathy (DR) disease recognition and classification. Our model leverages the power of CNN architectures: Inception V3 and VGG16 models by combining their strengths to cater to exact requirements. VGG16 model efficiently captures fine features and wide-ranging features such as textures and edges, crucial for classifying initial signs of DR. Similarly, Inception V3’s architecture is proficient at detecting multiscale patterns, providing an extensive setting for shaping the occurrence of more complex DR severity stages. Our deep hybrid model allows the extraction of various appearance features in retinal images, which can better assist the classification and detection of DR. Our proposed model evaluated on diverse datasets, including EyePACS1 and APTOS2019, demonstrating confident performance of 99.63% accuracy in classifying the DR severity levels on EyePACS1 dataset, while 98.70% accuracy on the APTOS2019 dataset, indicating that our proposed deep hybrid model well distinguished different stages and highly efficient in DR detection. This model helps clinicians and medical experts to classify and identify diabetic retinopathy DR stages and severity levels early. This automatic system helps to manage and treat the patient more effectively and introduces timely treatment.
Keywords
retinal images
diabetic retinopathy (DR)
deep hybrid model
Inception V3
VGG16
Data Availability Statement
Data will be made available on request.
Funding
This work was supported without any funding.
Conflicts of Interest
The authors declare no conflicts of interest.
Ethical Approval and Consent to Participate
Not applicable.
Cite This Article
APA Style
Shazia, A., Dahri, F. H., Ali, A., Adnan, M., Laghari, A. A., & Nawaz, T. (2024). Automated Early Diabetic Retinopathy Detection Using a Deep Hybrid Model. IECE Transactions on Emerging Topics in Artificial Intelligence, 1(1), 71–83. https://doi.org/10.62762/TETAI.2024.305743
Publisher's Note
IECE stays neutral with regard to jurisdictional claims in published maps and institutional affiliations.
Rights and permissions
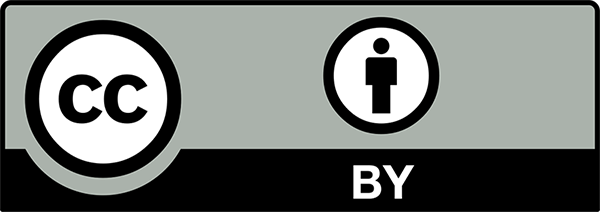
Copyright © 2024 by the Author(s). Published by Institute of Emerging and Computer Engineers. This article is an open access article distributed under the terms and conditions of the Creative Commons Attribution (CC BY) license (
https://creativecommons.org/licenses/by/4.0/), which permits use, sharing, adaptation, distribution and reproduction in any medium or format, as long as you give appropriate credit to the original author(s) and the source, provide a link to the Creative Commons licence, and indicate if changes were made.