Abstract
In this paper, a novel fast object detection framework is introduced, designed to meet the needs of real-time applications such as autonomous driving and robot navigation. Traditional processing methods often trade off between accuracy and processing speed. To address this issue, a hybrid data representation method is proposed that combines the computational efficiency of voxelization with the detail capture capability of direct data processing to optimize overall performance. The detection framework comprises two main components: a Rapid Region Proposal Network (RPN) and a Refinement Detection Network (RefinerNet). The RPN is used to generate high-quality candidate regions, while the RefinerNet performs detailed analysis on these regions to improve detection accuracy. Additionally, a variety of network optimization techniques have been implemented, including lightweight network layers, network pruning, and model quantization, to increase processing speed and reduce computational resource consumption. Extensive testing on the KITTI and NEXET datasets has proven the effectiveness of this method in enhancing the accuracy of object detection and real-time processing speed. The experimental results show that, compared to existing technologies, this method performs exceptionally well across multiple evaluation metrics, especially in meeting the stringent requirements of real-time applications in terms of processing speed.
Data Availability Statement
Data will be made available on request.
Funding
This work was supported without any funding.
Conflicts of Interest
The author declare no conflicts of interest.
Ethical Approval and Consent to Participate
Not applicable.
Cite This Article
APA Style
Wang, S.(2024). Real-Time Object Detection Using a Lightweight Two-Stage Detection Network with Efficient Data Representation. IECE Transactions on Emerging Topics in Artificial Intelligence, 1(1), 17–30. https://doi.org/10.62762/TETAI.2024.320179
Publisher's Note
IECE stays neutral with regard to jurisdictional claims in published maps and institutional affiliations.
Rights and permissions
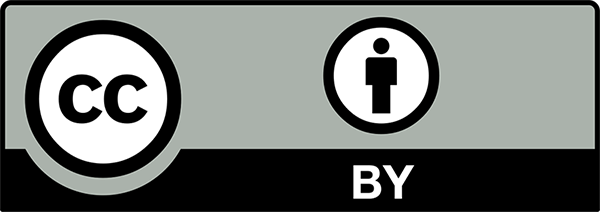
Copyright © 2024 by the Author(s). Published by Institute of Emerging and Computer Engineers. This article is an open access article distributed under the terms and conditions of the Creative Commons Attribution (CC BY) license (
https://creativecommons.org/licenses/by/4.0/), which permits use, sharing, adaptation, distribution and reproduction in any medium or format, as long as you give appropriate credit to the original author(s) and the source, provide a link to the Creative Commons licence, and indicate if changes were made.