Abstract
With the increasing global focus on renewable energy and the growing proportion of renewable power in the energy mix, accurate forecasting of renewable power demand has become crucial. This study addresses this challenge by proposing a multimodal information fusion approach that integrates time series data and textual data to leverage complementary information from heterogeneous sources. We develop a hybrid predictive model combining CNN and Bi-GRU architectures. First, time series data (e.g., historical power generation) and textual data (e.g., policy documents) are preprocessed through normalization and tokenization. Next, CNNs extract spatial features from both data modalities, which are fused via concatenation. The fused features are then fed into a Bi-GRU network to capture temporal dependencies, ultimately forming a robust CNN-Bi-GRU model. Comparative experiments with ARIMA, standalone GRU, and EEMD-ARIMA (a hybrid model combining ensemble empirical mode decomposition with ARIMA) demonstrate the superiority of our approach in both short- and long-term forecasting tasks on the same dataset. This research offers a potential framework to enhance renewable power demand prediction, supporting the industry’s sustainable growth and practical applications.
Keywords
multimodal information fusion
renewable electricity demand forecasting
CNN
Bi-GRU
predictive performance
Data Availability Statement
Data will be made available on request.
Funding
This work was supported without any funding.
Conflicts of Interest
The authors declare no conflicts of interest.
Ethical Approval and Consent to Participate
Not applicable.
Cite This Article
APA Style
Zhao, S., Xu, Z., Zhu, Z., Liang, X., Zhang, Z., & Jiang, R. (2025). Short and Long-Term Renewable Electricity Demand Forecasting Based on CNN-Bi-GRU Model. IECE Transactions on Emerging Topics in Artificial Intelligence, 2(1), 1–15. https://doi.org/10.62762/TETAI.2024.532253
Publisher's Note
IECE stays neutral with regard to jurisdictional claims in published maps and institutional affiliations.
Rights and permissions
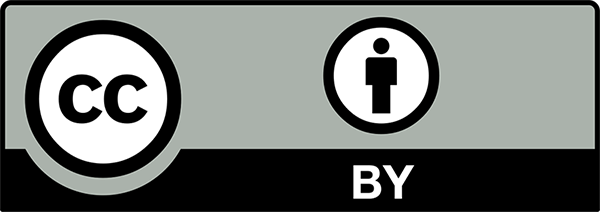
Copyright © 2025 by the Author(s). Published by Institute of Emerging and Computer Engineers. This article is an open access article distributed under the terms and conditions of the Creative Commons Attribution (CC BY) license (
https://creativecommons.org/licenses/by/4.0/), which permits use, sharing, adaptation, distribution and reproduction in any medium or format, as long as you give appropriate credit to the original author(s) and the source, provide a link to the Creative Commons licence, and indicate if changes were made.