Abstract
This paper proposes an improved object detection algorithm based on a dynamically deformable convolutional network (D-DCN), aiming to solve the multi-scale and variability challenges in object detection tasks. First, we review traditional methods in the field of object detection and introduce the current research status of improved methods based on multi-scale and variability convolutional neural networks. Then, we introduce in detail our proposed improved algorithms, including an improved feature pyramid network and a dynamically deformable network. In the improved feature pyramid network, we introduce a multi-scale feature fusion mechanism to better capture target information at different scales. In dynamically deformable networks, we propose dynamic offset calculations and dynamic convolution operations to achieve dynamic adaptation to the target shape and pose. We also validate our method by conducting experiments on the datasets KITTI and Caltech. Finally, we design a comprehensive loss function that considers both location localization error and category classification error to guide model training. Experimental results show that our improved algorithm achieves significant performance improvements in target detection tasks, with higher accuracy and robustness compared with traditional methods. Our work provides an effective method to solve the multi-scale and variability challenges in target detection tasks and has high practical value and prospects for general application.
Data Availability Statement
Data will be made available on request.
Funding
This work was supported without any funding.
Conflicts of Interest
The authors declare no conflicts of interest.
Ethical Approval and Consent to Participate
Not applicable.
Cite This Article
APA Style
Yang, J., & Gapar, Y. (2024). Improved Object Detection Algorithm Based on Multi-scale and Variability Convolutional Neural Networks. IECE Transactions on Emerging Topics in Artificial Intelligence, 1(1), 31-43. https://doi.org/10.62762/TETAI.2024.115892
Publisher's Note
IECE stays neutral with regard to jurisdictional claims in published maps and institutional affiliations.
Rights and permissions
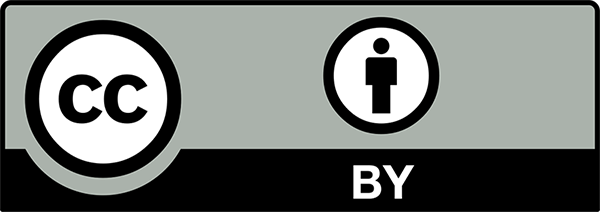
Copyright © 2024 by the Author(s). Published by Institute of Emerging and Computer Engineers. This article is an open access article distributed under the terms and conditions of the Creative Commons Attribution (CC BY) license (
https://creativecommons.org/licenses/by/4.0/), which permits use, sharing, adaptation, distribution and reproduction in any medium or format, as long as you give appropriate credit to the original author(s) and the source, provide a link to the Creative Commons licence, and indicate if changes were made.