Abstract
Abnormal fluctuations in financial markets may signal significant risks or market manipulation, so efficient time series anomaly detection methods are crucial for risk management. However, traditional statistical methods (e.g., ARIMA, GARCH) are difficult to adapt to the nonlinear and multi-scale characteristics of financial data, while single deep learning models (e.g., LSTM, Transformer) have limitations in capturing long-term trends and short-term fluctuations. In this paper, we propose WaveLST-Trans, a financial time series anomaly detection model based on the combination of wavelet transform (WT), LSTM and Transformer. The model first uses wavelet transform to perform multi-scale decomposition, extracts low-frequency trend and high-frequency fluctuation features, and feeds them into LSTM (to learn the long-term dependence) and Transformer (to capture local mutations) respectively, and finally integrates the multi-scale information through the feature fusion layer, which improves the detection accuracy and robustness. The experiments are conducted on Binance (cryptocurrency market) and S&P 500 (stock market) datasets, and the results show that WaveLST-Trans mostly outperforms the mainstream models in terms of F1-score, recall, and precision, and improves the detection performance by 3% and 10% in high-frequency market and long-term trend market, respectively. This study provides a more accurate and stable anomaly detection method for financial market risk management, which can be widely used in market regulation, quantitative trading and financial risk control, helping to improve the security and stability of the financial system.
Data Availability Statement
Data will be made available on request.
Funding
This work was supported without any funding.
Conflicts of Interest
Tian Su is an employee of Meta Platforms Inc., Seattle, WA 98109, United States.
Ethical Approval and Consent to Participate
Not applicable.
Cite This Article
APA Style
Su, T., Li, R., Liu, B., Liang, X., Yang, X., & Zhou, Y. (2025). Anomaly Detection and Risk Early Warning System for Financial Time Series Based on the WaveLST-Trans Model. IECE Transactions on Emerging Topics in Artificial Intelligence, 2(2), 68–80. https://doi.org/10.62762/TETAI.2025.191759
Publisher's Note
IECE stays neutral with regard to jurisdictional claims in published maps and institutional affiliations.
Rights and permissions
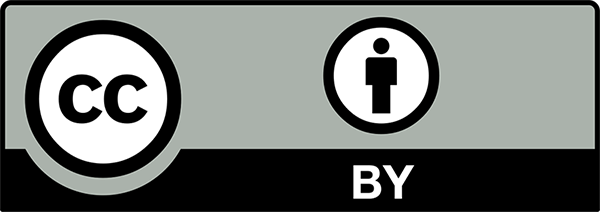
Copyright © 2025 by the Author(s). Published by Institute of Emerging and Computer Engineers. This article is an open access article distributed under the terms and conditions of the Creative Commons Attribution (CC BY) license (
https://creativecommons.org/licenses/by/4.0/), which permits use, sharing, adaptation, distribution and reproduction in any medium or format, as long as you give appropriate credit to the original author(s) and the source, provide a link to the Creative Commons licence, and indicate if changes were made.