IECE Transactions on Machine Intelligence | Volume 1, Issue 1: 6-16, 2025 | DOI: 10.62762/TMI.2025.796490
Abstract
Traditional centralized machine learning approaches for IoT botnet detection pose significant privacy risks, as they require transmitting sensitive device data to a central server. This study presents a privacy-preserving Federated Learning (FL) approach that employs Federated Averaging (FedAvg) to detect prevalent botnet attacks, such as Mirai and Gafgyt, while ensuring that raw data remain on local IoT devices. Using the N-BaIoT dataset, which contains real-world benign and malicious traffic, we evaluated both the IID and non-IID data distributions to assess the effects of decentralized training. Our approach achieved 97.5% accuracy in IID and 95.2% in highly skewed non-IID scenarios, clos... More >
Graphical Abstract
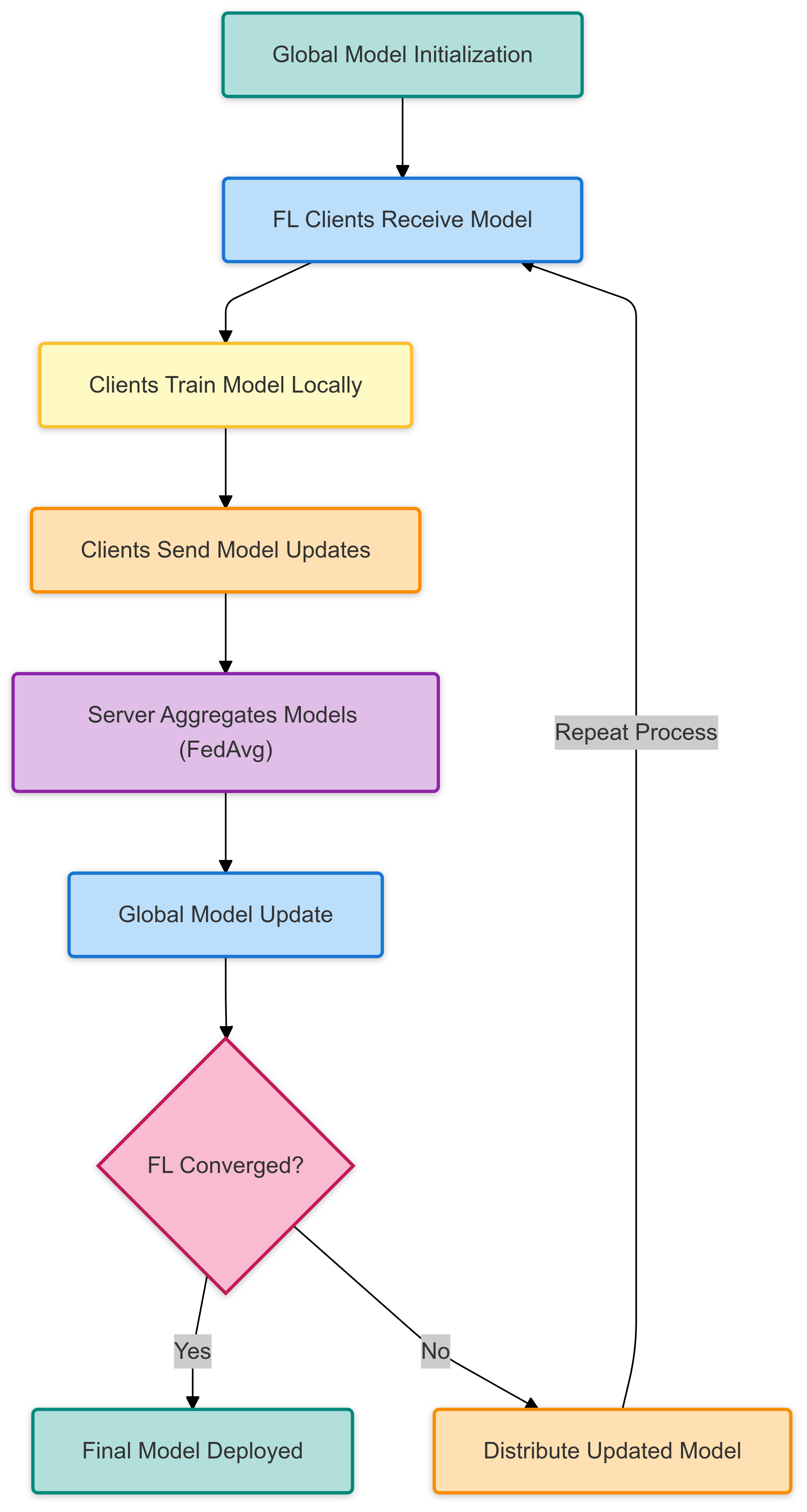