Abstract
As a carrier of genetic information, the precise design and optimization of nucleic acid (DNA or RNA) sequences are of critical importance for the realization of specific biological functions. From synthesizing genes to designing novel nucleic acid drugs, from constructing efficient expression vectors to modifying microbial metabolic pathways, all are inseparable from the fine regulation of nucleic acid sequences. The primary purpose of nucleic acid sequence design is to generate new sequences tailored to specific requirements for gene expression, function prediction, drug development, and other applications. In this paper, we first review the theoretical basis of nucleic acid sequence design, followed by an overview of current research methods for nucleic acid sequence design. Traditional nucleic acid sequence design methods rely on manual experience and experimentation, and although some progress has been made in the past decades, they still suffer from high cost, long time, and low efficiency in most cases. Therefore, optimizing nucleic acid sequences to improve their performance and stability has become particularly important. In recent years, artificial intelligence technology has provided a new direction for the design and optimization of nucleic acid sequences, opening up new possibilities for more efficient and accurate design methods. These methods include traditional rule-based nucleic acid sequence optimization approaches as well as AI-driven optimization methods for nucleic acid sequence generation. This review systematically examines the latest advancements in both traditional and AI-driven nucleic acid sequence design methods and analyzes the technical details, strengths, and limitations of each application. Finally, the article discusses the current challenges and future development directions of nucleic acid sequence design.
Keywords
nucleic acid sequence design
machine learning
optimization methods
generative models
heuristic algorithms
large language models
nucleic acid structure prediction
Data Availability Statement
Data will be made available on request.
Funding
This work was supported in part by the 111 Project under Grant D23006; in part by the National Natural Science Foundation of China under Grant 62272079; in part by the National Foreign Expert Project of China under Grant D20240244; in part by the Natural Science Foundation of Liaoning Province under Grant 2024-MS-212; in part by the Scientific Research Project of Liaoning Provincial Department of Education under Grant LJ222411258005; in part by the LiaoNing Revitalization Talent Program under Grant XLYC2403039; in part by the Artificial Intelligence Innovation Development Plan Project of Liaoning Province under Grant 2023JH26/10300025; in part by the Dalian Outstanding Young Science and Technology Talent Support Program under Grant 2022RJ08; in part by the Dalian Major Projects of Basic Research under Grant 2023JJ11CG002; in part by the Interdisciplinary Project of Dalian University under Grant DLUXK-2024-YB-001; in part by the Joint plan of Liaoning Province science and technology plan under Grant 2024JH2/102600064 and Grant 2024-MSLH-009.
Conflicts of Interest
The authors declare no conflicts of interest.
Ethical Approval and Consent to Participate
Not applicable.
Cite This Article
APA Style
Yang, T., Han, M., Wen, X., & Zheng, Y. (2025). Advances in Intelligent Design and Optimization Methods for Nucleic Acid Sequences. Journal of Artificial Intelligence in Bioinformatics, 1(1), 12–29. https://doi.org/10.62762/JAIB.2025.194547
Publisher's Note
IECE stays neutral with regard to jurisdictional claims in published maps and institutional affiliations.
Rights and permissions
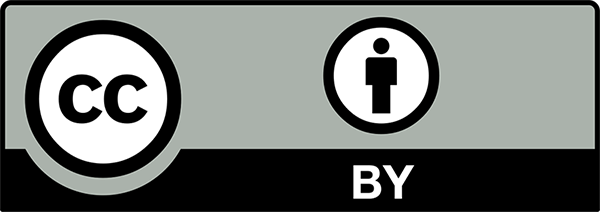
Copyright © 2025 by the Author(s). Published by Institute of Emerging and Computer Engineers. This article is an open access article distributed under the terms and conditions of the Creative Commons Attribution (CC BY) license (
https://creativecommons.org/licenses/by/4.0/), which permits use, sharing, adaptation, distribution and reproduction in any medium or format, as long as you give appropriate credit to the original author(s) and the source, provide a link to the Creative Commons licence, and indicate if changes were made.